“Having access to EmojiNet can help computers to interpret the meaning of emoji.” -Sanjaya Wijeratne
Sorry Freud. But when it come to emoji, a cigar isn’t always a cigar (if there was, in fact, a cigar emoji).
In 2016, Wright State University computer science Ph.D student Sanjaya Wijeratne was working to identify at-risk youth using social media posts. While following twitter accounts of the gang-affiliated youth he began to notice unique emoji uses. Tweets expressing anger towards law enforcement, for example. frequently featured gun and police officer emoji.
But the most frequently used emoji? The gas pump. “We were surprised at first, but by looking at tweets using this emoji we discovered they mainly use it when talking about drugs, because “gas” is a slang term for marijuana.”
“That’s when it first struck me that emojis could take different meanings. I saw people using the same emoji in different contexts with different meanings, and I wanted to find a resource that lists all the meanings for a particular emoji.”
Flash forward to EmojiNet, the first machine-readable sense inventory for emoji, developed by Sanjaya and his group at Kno.e.sis, a project of Wright State University. Since Sanjaya’s gas pump epiphany, he and his team composed of fellow graduate student Lakshika Balasuriya, and advisors Prof. Amit Seth and Dr. Derek Doran, published three papers on their work, and are currently developing applications to help computers automatically infer emoji meaning.
Semantic Search
Sanjaya’s research on emoji is closely related to the concept of semantic search, a growing area of study and application related to database and web searching. Rather than searching by keyword, semantic search considers the searcher’s intent and the context in which search terms are used to improve accuracy, and generate better results.
Semantic search has huge implications for search engine optimization, as users have begun to lengthen search queries to gain more precise results. Google recently reorganized its search algorithm to consider context and intent, prioritizing the “understanding” phase of search over “achieving”, “filtering and clustering” and “ranking”.
So how do we figure know what a word means in a given context? For us, it’s mostly subconscious. For computers? Not so much. One of the cornerstones of semantic search is word-sense disambiguation. Word-sense disambiguation is a long-term with a simple meaning: determining the meaning of a word used in a particular sense, when the word has multiple possible meanings.
So… back to emoji.
Making an Emoji Sense Inventory
Sanjaya and his team are developing programs to help computers detect and understand multiple meanings of emoji. In other words, they study emoji sense disambiguation . An easy way to understand emoji sense disambiguation is to compare the results of two open source emoji databases.
Emoji Dictionary and Emojipedia are both unicode-based emoji databases, with starkly different purposes. Emojipedia results contain static definitions of each emoji, followed each rendering of the emoji, statistics, and links to related emoji. In other words, Emojipedia is the emoji equivalent of a dictionary. An Emojipedia search for “folded hands”, for example, displays the following result:
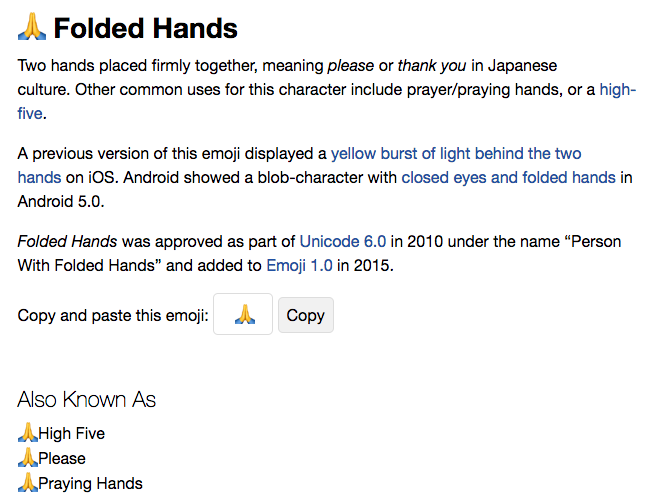
Emoji Dictionary, on the other hand, presents fluid and contextual search results, based on crowd-sourced definitions, nouns, verbs, adjective, and adverbs. Like Urban Dictionary, Emoji Dictionary explores multiple meaning of emoji based on content and intent. Compare the previous “folded” definition to Emoji Dictionary’s results:
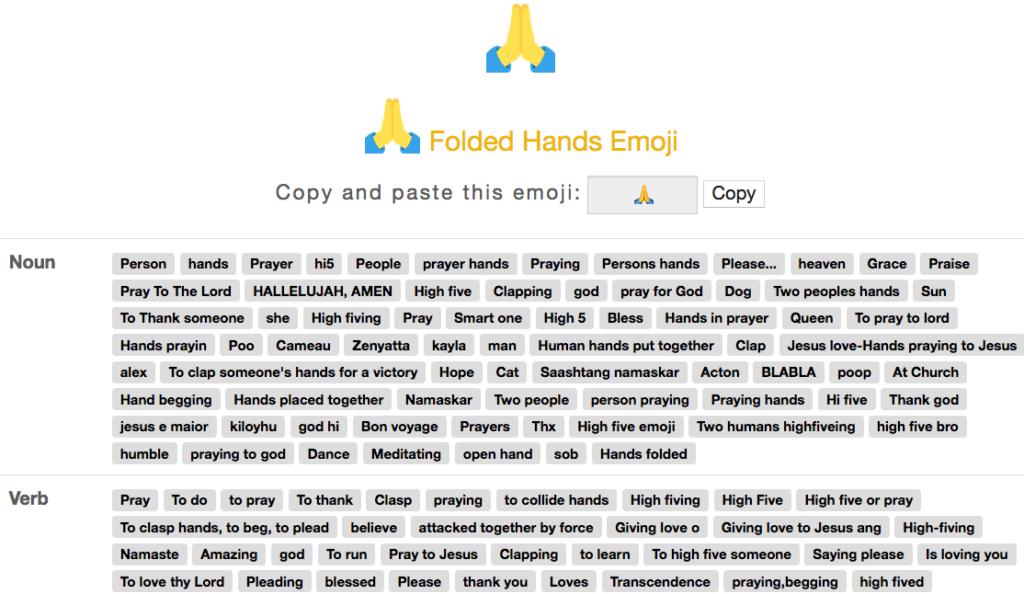
Whereas Emojipedia gives a short, concise definition of the “folded hands” emoji, Emoji Dictionary, on the other hand, provides a flexible list nouns, verbs, adjective, adverbs and examples, indicative of user intent and context.
Sanjaya and his team’s goal was to create a machine readable “sense inventory” of emoji, so computers could understand the semantic meaning of an emoji in a particular sentence. Upon discovery of several open source emoji databases online, including Unicode Consortium, Emoji Dictionary, iEmoji and Emojipedia. the team extracted contextual and part of speech meaning (noun, verb, adjective, etc.) of each emoji from Emoji Dictionary, then integrated the information with Babelnet, a multilingual semantic dictionary and database dedicated to word-sense disambiguation.
Thus was born EmojiNet.
EmojiNet
EmojiNet is the first machine-readable sense inventory for emoji, linking emoji represented as Unicode with English language meanings from the Web. By integrating online emoji databases with Babelnet, EmojiNet allows users to infer sense definitions from communication using emoji. For a better picture, check out EmojiNet results for “folded hands“.
“Emoji plays a huge role in deciding the sentiment or emotion of a particular text. But in certain platforms, and certain carriers, emoji are shown differently. If you look at how people use face with tears of joy emoji, they mostly use it to express happiness, but sometimes it is associated with sadness too. But if we build an application that can disambiguate emoji meanings, we’d be able to tell the meanings of an emoji in a text context, that would have a direct impact on applications such as sentiment and emotion analysis.”
The team is also focused on measuring emoji similarity by determining pairs of emojis frequently used together to enhance semantic search, and develop applications to automatically process and infer meaning from emoji based communication. These applications could improve web and database search, sentiment analysis, and interface design. Grouping similar emoji together on mobile keyboards, for example, could make texting with emoji less time consuming.
What’s Next?
Sanjaya and his team’s attempts to promote his team’s paper were frustrated by the current administration’s immigration policy. When the group’s paper was accepted at the International AAAI Conference on Web and Social Media, Sanjaya was unable to attend . A Sri Lankan native, Sanjaya is on a student visa. “We wanted to promote our paper at IABC , but If I left the country, I’d have to return to Sri Lanka to reapply.” With the current administration’s immigration policy, this is not a chance Sanjaya wanted to take.
Despite earlier frustration, EmojiNet is beginning to get attention from tech companies. Recently, data science platform Kaggle inducted EmojiNet as a featured dataset. EmojiNet is also in the process of organizing an emoji prediction challenge with Google, Microsoft, and Kaggle using EmojiNet data.
Sanjaya is excited to unleash EmojiNet’s potential. “I would argue that having access to EmojiNet can help computers to interpret the meaning of emoji. We want to improve tasks such as sentiment and emotion analysis with this information.”
To learn more about EmojiNet, emoji similarity, and emoji sense disambiguation, check out these articles!